Before any AI solution can bring real value, one step must come first: data collection for AI.
It’s not the flashiest part of the journey — but without clean, relevant, and well-organized data, even the most advanced models can’t deliver reliable results. Poor data leads to poor predictions, wasted resources, and AI tools that simply don’t work as expected.
Whether you’re planning to automate internal processes or launch customer-facing tools, your success depends on what you feed models — and that starts with a solid data collection strategy.
🪼 I’m Maj — a jellyfish generated by AI, here to guide you through the depths of this topic. Together, we’ll explore why data collection for AI is the foundation of implementation, how to approach it strategically, and what to avoid so your project doesn’t sink before it starts.
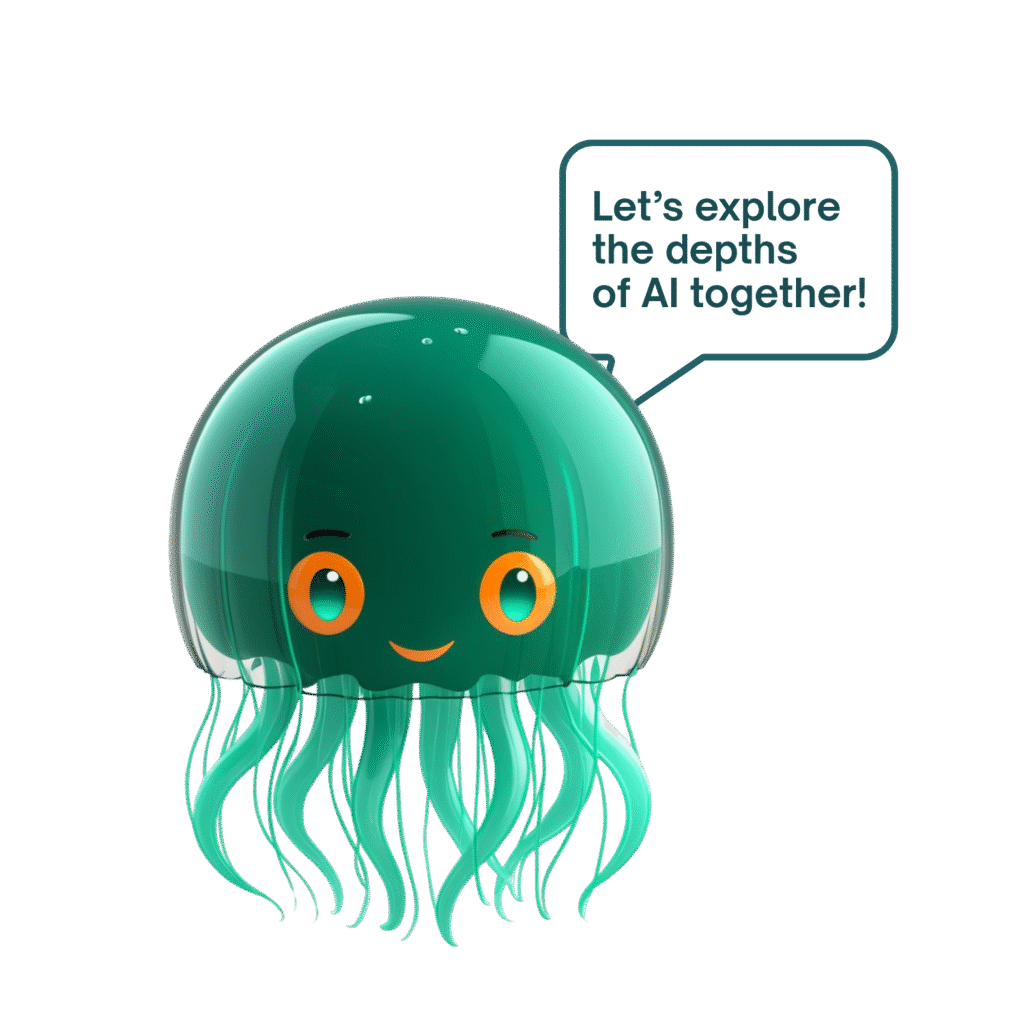
Let’s explore the depths of AI together!
➡️ If you’re interested in learning more about Maj the Jellyfish and the AI Ocean, read the article ‘What Can a Jellyfish Teach You About Artificial Intelligence? Meet Maj!’
🌊 Surface 1: Why data collection is the first step?
Before you dive into AI implementation, there’s one essential layer beneath the surface: data collection for AI. Without it, your AI initiative is like navigating without a map — ambitious, but likely to drift off course.
💡Here’s why it matters so much:
🔎 Poor-quality data leads to poor-quality outputs — no matter how advanced the model is.
🔎 Without diverse, representative input, AI can reinforce harmful patterns or miss the full picture.
🔎 Good data accelerates everything. Clean, structured, relevant data shortens implementation time and improves outcomes.
🔎 You can’t fix data later. If collection is skipped or done poorly, retrofitting quality later is expensive and inefficient.
📌 One of the most common mistakes in AI implementation is skipping or rushing through the data stage.
But data collection for AI isn’t optional — it’s the foundation everything else depends on.
✅ AI learns by example
It needs patterns, context, and scale — and all of that comes from the data you give it.
🔴 Common problems with data in organizations:
- Data is scattered across departments or tools.
- No one has verified how recent or accurate it is.
- It’s not aligned with the goals of the AI solution.
- There’s no audit or structure — just assumptions.
✅ What to do?
Before implementing tools or models, it’s important to begin with a review of the following:
- ✔️ What data is already available?
- ✔️ Whether the data is recent, accurate, and accessible.
- ✔️ Whether it reflects the real-world problem the AI solution is meant to address.
Clear documentation at this stage is essential. Even a simple data readiness assessment can help avoid delays, errors, and unnecessary effort later on.
✅ Need help with this step?
We help teams audit and structure their data collection for AI — click here to contact us!
🌊 Surface 2: What makes data “good enough” for AI implementation?
Not all data is created equal — especially when it comes to AI.
You might already have tons of spreadsheets, reports, or logs.
❓But the question isn’t just “Do we have data?” — it’s “Is our data usable for AI?”
🔎 Why it matters?
AI models need data that is:
- Relevant – aligned with the goal of the AI use case.
- Structured – clean, labeled, and easy to interpret.
- Consistent – similar inputs yield similar formats.
- Complete – no major gaps or missing fields.
Without these traits, even a powerful AI model will underperform — or worse, produce unreliable or biased results.
✅ What to do?
✔️ Define your AI use case first — then check if the data supports it.
✔️ Audit sample data: Is it clean? Consistent? Complete?
✔️ Use lightweight preprocessing tools to improve quality (many are free).
✔️ Involve domain experts to validate what “quality” means in your context.
🌊 Surface 3: Common data collection mistakes that undermine AI implementation
🔎 Data collection for AI is often seen as the easiest part of the process — but that assumption is exactly what leads to costly mistakes later on.
It’s not always about missing data. More often, it’s subtle issues in how data is collected, stored, or labeled that quietly sabotage AI implementation efforts.
🔎 Why this matters?
Even when organizations invest in data collection for AI, these frequent issues can compromise the results:
- ❌ No clear objective behind what’s collected.
- ❌ Siloed or scattered data across tools and teams.
- ❌ Missing essential context (like user behavior or time stamps).
- ❌ Inconsistent structures and formats.
- ❌ Poor or unreliable labeling practices.
✅ What to do?
✔️ Defining what “useful data” looks like for your specific goal.
✔️ Aligning formats, field structures, and naming conventions across systems.
✔️ Applying tagging and categorization that supports downstream models.
✔️ Reviewing and cleaning datasets before moving to modeling.
➡️ Curious “How to start with AI Implementation?” Read the full article here!
🌊 Surface 4: The role of human expertise in data collection for AI
It’s a common misconception that AI can fully manage data on its own.
In reality, data collection for AI is not purely a technical task — it requires human oversight and informed decision-making at every stage.
🔎 Why this matters?
📌 AI can only learn what it’s fed — and if the people feeding it don’t understand the domain or the goals, the entire implementation suffers.
➡️ Here’s where human expertise is essential:
- 🧠 Defining what should be measured.
- 🧹 Spotting biased, duplicated, or irrelevant data early.
- 🧭 Providing business context AI can’t infer.
- 🏷️ Ensuring correct labeling and categorization based on real-world meaning.
- 📊 Interpreting results and adjusting collection practices accordingly.
✅ To improve data collection for AI with human oversight:
- Involve subject-matter experts in defining and reviewing datasets.
- Use annotation tools that allow human feedback.
- Build workflows where data teams and domain teams regularly align.
- Treat data governance as a shared, ongoing responsibility.
🌊 Surface 5: Data privacy and ethics in AI data collection
📌 When it comes to data collection for AI, there’s a critical issue you cannot overlook: privacy and ethics.
As AI becomes more powerful, the responsibility for handling data ethically is greater than ever.
Whether it’s customer data, employee data, or proprietary data, how it’s collected, stored, and used has massive implications for your organization’s reputation and legal standing.
🔎 To ensure responsible data collection for AI, consider these best practices:
- ✅ Stay compliant with data protection laws (GDPR, CCPA, etc.).
- ✅ Regularly audit your data collection processes for privacy risks.
- ✅ Ensure transparency with your data subjects (e.g., consent for data usage).
- ✅ Implement strong data encryption and security protocols.
- ✅ Consider ethical implications of the data and avoid collecting unnecessary personal information.
❇️❇️ If assistance is needed in preparing the data collection process for AI implementation, feel free to contact us (click here)!